Cloud traffic pbkp_rediction based on fuzzy ARIMA model with low dependence on historical data
Abstract:
Traffic pbkp_rediction with high accuracy has become a vital and challenging issue for resource management in cloud computing. It should be noted that one of the prominent factors in resource management is accurate traffic pbkp_rediction based on a few data points and within a short time period. The autoregressive integrated moving average (ARIMA) model is a suitable model to pbkp_redict traffic in short time periods. However, it requires a massive amount of historical data to achieve accurate results. On the other hand, the fuzzy regression model is adequate for pbkp_rediction using less historical data. Aforementioned by these considerations, in this paper, a combination of ARIMA and fuzzy regression called fuzzy autoregressive integrated moving average (FARIMA) is used to forecast traffic in cloud computing. Besides, we adopt the FARIMA model by using the sliding window, called SOFA, concept to determine models with higher pbkp_rediction accuracy. Accuracy comparison of these models based on the root means square error and coefficient of determination demonstrates that SOFA is about 5.4 and 0.009, respectively, which is the superior model for traffic pbkp_rediction.
Año de publicación:
2022
Keywords:
Fuente:
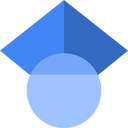
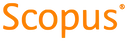
Tipo de documento:
Article
Estado:
Acceso restringido
Áreas de conocimiento:
- Análisis de datos
- Algoritmo
- Modelo estadístico
Áreas temáticas:
- Ciencias de la computación