Cold-Start Promotional Sales Forecasting through Gradient Boosted-Based Contrastive Explanations
Abstract:
Multiple Machine Learning solutions in Industry exist where interpretability is required. In retail, this is especially important when dealing with cold-start forecasting of promotional sales. In the planning phase of these promotions, retailers produce sales predictions that are scrutinised by both forecasting experts and managers. In this paper, we combine the predictive benefits of Gradient Boosted Decision Trees regressors and the interpretability of contrastive explanations. These are implicitly generated by the manner we shape data. Our method presents the cold-start forecasts in relation to the observed promotional sales of other products, which we call neighbours. They are selected based on a measure of closeness to the predicted promotion, which is derived from the variable importance calculated during the training of the regressors. With this information, the expert reviewer adjusts the cold-start prediction by simply varying the contribution of the neighbours. To validate our results we test our method on a surrogate model, as well as on real-market data. The results on the surrogate model demonstrate that our method is able to accurately identify the features that contribute to sales and then select the closest neighbours to produce a contrastive explanation. The results on real-market data also show that the proposed method performs at a similar level to widespread methods such as conventional CatBoost, NGBoost or AutoGluon, and has the advantage of providing interpretability.
Año de publicación:
2020
Keywords:
- Cold-start forecasting
- contrastive explanations
- retail promotions
- interpretable ML (iML)
- supply chain
Fuente:
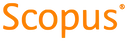
Tipo de documento:
Article
Estado:
Acceso abierto
Áreas de conocimiento:
- Aprendizaje automático
Áreas temáticas de Dewey:
- Funcionamiento de bibliotecas y archivos

Objetivos de Desarrollo Sostenible:
- ODS 12: Producción y consumo responsables
- ODS 8: Trabajo decente y crecimiento económico
- ODS 9: Industria, innovación e infraestructura
