Collaborative filtering optimization based on matrix factorization through the relevance of user preferences
Abstract:
Collaborative filtering based on matrix factorization has become the reference method for the recommendation of products or services due to the high precision of recommendations it generates. These methods factoring the matrix that contains user preferences into two new matrices that symbolize the properties of users and products or services in a space of latent factors. This paper proposes a new matrix factorization model weighted according to the users’ preference over products or services. The experimental results carried out on the datasets of MovieLens 100K, MovieLens 1M and Netflix demonstrate a clear improvement in terms of quality of pbkp_redictions and recommendations compared to other matrix factorization techniques.
Año de publicación:
2020
Keywords:
- matrix factorization
- Gradient descent
- Recommendation system
- COLLABORATIVE FILTERING
Fuente:
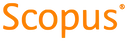
Tipo de documento:
Article
Estado:
Acceso restringido
Áreas de conocimiento:
- Aprendizaje automático
- Algoritmo
- Algoritmo
Áreas temáticas:
- Funcionamiento de bibliotecas y archivos