Combining Filter-Based Feature Selection Methods and Gaussian Mixture Model for the Classification of Seismic Events from Cotopaxi Volcano
Abstract:
This paper proposes an exhaustive evaluation of five different filter-based feature selection methods in combination with a Gaussian mixture model classifier for the classification of long-period (LP) and volcano-Tectonic (VT) seismic events recorded at Cotopaxi volcano in Ecuador. The experimentation included both exploring and ranking search spaces of seismic-signal-based features, and selecting subsets of optimal features for event classification. The evaluation was carried out by using an experimental dataset formed by 587 LP and 81 VT feature vectors, each composed of 84 statistical, temporal, spectral, and scale-domain features extracted from the original seismic signals. The best result in accuracy, precision, recall, and processing time for LP seismic event classification was obtained by using the Chi2 discretization method with five features, achieving 95.62%, 99.08%, 95.94%, and 3.7 ms, respectively, whereas for VT seismic event classification, the uFilter method with five features reached the scores of 96.71%, 85.23%, 96.00%, and 4.1 ms, respectively. For the classification of both seismic events simultaneously, the uFilter method with five features yielded 96.70%, 97.77%, 96.7%, and 4.1 ms, respectively. According to the Wilcoxon statistical test, these classification schemes provide competitive seismic event classification, while reducing the required processing time.
Año de publicación:
2019
Keywords:
- Feature selection methods
- Gaussian mixture model (GMM) classifier
- Redundancy analysis
- Seismic Events Classification
Fuente:
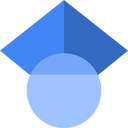
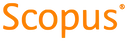
Tipo de documento:
Article
Estado:
Acceso restringido
Áreas de conocimiento:
- Sismología
- Sismología
- Aprendizaje automático
Áreas temáticas:
- Ciencias de la tierra
- Cultivos de huerta (horticultura)