Combining feature engineering and feature selection to improve the pbkp_rediction of methionine oxidation sites in proteins
Abstract:
Methionine is a proteinogenic amino acid that can be post-translationally modified. It is now well established that reactive oxygen species can oxidise methionine residues within living cells. For a long time, it has been thought that such a modification represents merely an inevitable damage derived from aerobic metabolism. However, several authors have begun to contemplate a possible role for this methionine modification in cell signalling. During the last years, a number of proteomic studies have been carried out with the purpose of detecting proteins containing oxidised methionines. Although these proteomic works allow to pinpoint those methionines being oxidised, they are also arduous, expensive and time-consuming. For these reasons, computational approaches aimed at pbkp_redicting methionine oxidation sites in proteins become an appealing alternative. In the current work, we address methionine oxidation pbkp_rediction by combining computational intelligence methods with feature engineering and feature selection techniques to improve the efficacy of several machine learning models, while reducing the number of input characteristics needed to get high accuracy rates. We compare random forests, support vector machines, neural networks and flexible discriminant analysis models. Random forests give the best AUC (0.8124 ± 0.0334) and accuracy rates (0.7590 ± 0.0551) by using only a reduced set of 16 characteristics. These results surpass the outcomes of previous works. In addition, we present an end-user script that has been developed to take a protein ID as an input and return a list with the oxidation state of all the methionine residues found in the analysed protein. Finally, to illustrate the applicability of this tool, we have selected the human α1 -antitrypsin protein as a case study. This protein was selected because it was not present among the set of proteins used to build up the pbkp_redictive models but the protein has been well characterised experimentally in terms of methionine oxidation. The pbkp_rediction returned by our script fully matches the empirical evidence. Out of the nine methionine residues found in this protein, our model pbkp_redicts the oxidation of only two of them, M351 and M358, which have been reported, on the base of mass spectrometry analyses, to be particularly susceptible to oxidation.
Año de publicación:
2020
Keywords:
- Methionine oxidation
- post-translational modification
- Pbkp_redictive computational model
- Protein pbkp_rediction
Fuente:
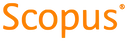
Tipo de documento:
Article
Estado:
Acceso restringido
Áreas de conocimiento:
- Proteína
Áreas temáticas:
- Ciencias de la computación
- Bioquímica
- Métodos informáticos especiales