Comparative Analysis of the Performance of Machine Learning Techniques Applied to Real and Synthetic Fraud-Oriented Datasets
Abstract:
One of the most critical resources today is information, an intangible asset that has become a vital research source. On many occasions, access to data becomes a complex and challenging task. For many organizations sharing information, it is often a risk in terms of security and privacy, especially if the data is sensitive. In response to this problem, synthetic data emerges as a valid alternative, generated by different methods and techniques from an original or real dataset, allowing sharing of information very close to reality. In this work, an experiment is carried out that allows validating the efficiency of synthetic versus real datasets by applying a model that pbkp_redicts possible fraud cases in a dataset based on machine learning algorithms LDA and Random Forest or Gradient Boosting. We compared the pbkp_rediction performance of our model over the real and synthetic datasets using metric ROC-AUC curves. Our results show a similar behavior among the data sets in our model, suggesting a promising path in the use of synthetic data sets for this kind of applications.
Año de publicación:
2022
Keywords:
- AUC-ROC
- Classification Methods
- Real and synthetic dataset
- Topic modeling
- Fraud
Fuente:
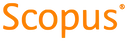
Tipo de documento:
Conference Object
Estado:
Acceso restringido
Áreas de conocimiento:
- Aprendizaje automático
- Ciencias de la computación
Áreas temáticas de Dewey:
- Métodos informáticos especiales
- Programación informática, programas, datos, seguridad
- Funcionamiento de bibliotecas y archivos