Comparative Study of Deep Learning Algorithms in the Detection of Phishing Attacks Based on HTML and Text Obtained from Web Pages
Abstract:
Phishing webpages are a type of cyber-attack whose objective is to try to deceive people through fraudulent web pages to harm its victims, commonly in an economical way. Every day new fraudulent web pages are created, so one of the standard methods to detect these recent attacks is the use of Artificial Intelligence algorithms based on the HTML content of a web page. Therefore, this work aims to perform a comparative analysis between Deep Learning algorithms to know if it is more effective to detect an attack, either using HTML code or the text obtained from this code. The content of a large text Dataset was obtained using Web Scraping techniques. Then the Deep Neural Network (DNN), Recurrent Neural Network (RNN), Convolutional Neural Network (CNN), and Recurrent Convolutional Neural Network (RCNN) algorithms were executed, feeding them first with HTML code and then with text. The average of the metrics obtained with HTML was 85%, and the overall metrics obtained for text averaged 84%. In conclusion, it is determined with this study that it makes no difference whether the algorithm is fed with HTML or text because when analyzing with text, the unnecessary features of HTML are eliminated, but simultaneously, the essential elements of HTML are also lost.
Año de publicación:
2023
Keywords:
- Text content
- HTML code content
- WEB SCRAPING
- Phishing
- deep learning
Fuente:
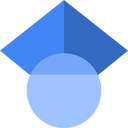
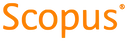
Tipo de documento:
Conference Object
Estado:
Acceso restringido
Áreas de conocimiento:
- Aprendizaje automático
- Ciencias de la computación
Áreas temáticas de Dewey:
- Ciencias de la computación

Objetivos de Desarrollo Sostenible:
- ODS 9: Industria, innovación e infraestructura
- ODS 17: Alianzas para lograr los objetivos
- ODS 8: Trabajo decente y crecimiento económico
