Comparative analysis of machine learning pbkp_rediction models of container ships propulsion power
Abstract:
Regulations on Greenhouse Gas (GHG) ship's emissions and air pollutant are becoming more restrictive. Therefore, a big effort is being put into ship efficiency discussion, specially on pbkp_redictive models related to route optimization, fuel consumption and air emissions. This paper compares machine learning pbkp_redictive algorithms, based on the following techniques: least-squares, decision trees and neural networks, to estimate ship propulsion power between two 8400 TEU container ships from the same series. Additionally, the influence of having a pbkp_redictive algorithm trained with data of its sister ships is invesitgated. The data used in this study were recorded from 2009 to 2014 reaching almost 290,000 entries. The results indicate that random forest regression model and decision trees ensemble models have the best fit for this purpose. It has also confirmed the feasibility of pbkp_redicting the delivered power of a ship having a machine learning algorithm feed with a sister ship information despite differences in the route and/or operating conditions.
Año de publicación:
2022
Keywords:
- Machine learning
- Propulsion power
- Pbkp_rediction models
- Container ships
- fuel consumption
- Gas emissions
- marine transport
Fuente:
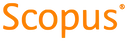
Tipo de documento:
Article
Estado:
Acceso restringido
Áreas de conocimiento:
- Simulación por computadora
- Aprendizaje automático
Áreas temáticas:
- Métodos informáticos especiales
- Otras ramas de la ingeniería
- Programación informática, programas, datos, seguridad