Comparing tag clustering algorithms for mining Twitter users' interests
Abstract:
This paper addresses the problem of mining users' interest from the vast, noise, unstructured and dynamic data generated on social media sites, taking Twitter as case study. The mining process uses different Natural Language Processing techniques to extract the relevant words from subscribers' tweets and applies cluster analysis over them. We evaluate the performance of three different tag clustering algorithms - PAM, Affinity Propagation and UPGMA- when considering the hyperlink structure of Wikipedia as external source for semantic closeness among words. We provide a solution which can be developed without any a-priori knowledge about the number and category of topics, neither a priori knowledge about the users we are applying the extraction for. This solution is based on using an unsupervised measure of the clustering quality (Silhouette width) to estimate the parameters of the cluster analysis. Finally, as human feedback is not as reliable as expected, we validate the approach by using Twitter hashtags - the implicit classifying method used by Twitter users to organise their tweets. © 2013 IEEE.
Año de publicación:
2013
Keywords:
Fuente:
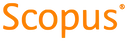
Tipo de documento:
Conference Object
Estado:
Acceso restringido
Áreas de conocimiento:
- Minería de datos
- Análisis de redes sociales
- Ciencias de la computación
Áreas temáticas:
- Funcionamiento de bibliotecas y archivos