Comparison of classical and machine-learning methods on spatio-temporal modeling of daily Ozone concentrations
Abstract:
Effective actions to mitigate air pollution require of availability of high-resolution observations. Low-cost sensor technologies have emerged as an affordable solution to cope with this deficiency. However, since low-cost sensors are built with low-cost materials, they are prone to errors, gaps, bias, and noise. These problems need to be solved before data can be used to support research or decision making. Addressing lack of reliability in low-cost sensor data is a complex challenge that is still under research over several lines (e.g. accuracy estimation of low-cost sensor data). Current approaches in this line involve modeling, bias-correction, and more recently, data fusion methods relying on high-resolution air quality computational models. Overall, accuracy estimation can be reduced to a modeling problem. The focus of this work is studying, testing, and comparing suitable approaches for handling point-referenced spatio-temporal sensor data, particularly classical spatial models, spatio-temporal models, and popular machine learning methods. Among these approaches, Bayesian hierarchical models have a special consideration given the attention they have drawn during the last fifteen years. The benchmark supporting this comparison study is a real-life dataset made up of daily ozone observations taken from the USA Environmental Protection Agency (EPA) and meteorological variables extracted from the NCEP/NCAR Reanalysis Project (NNRP). The main contributions of this work are: (1) a systematic comparison of three kinds of models, using a 10-fold cross-validation exercise; and (2) a feature engineering method to create covariates meant to harness spatially correlated observations of point-referenced sensor data.
Año de publicación:
2020
Keywords:
- Gaussian Process
- air pollution
- MACHINE-LEARNING
- sensor data
- spatio-temporal models
Fuente:
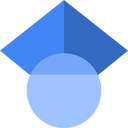
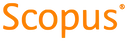
Tipo de documento:
Conference Object
Estado:
Acceso restringido
Áreas de conocimiento:
- Aprendizaje automático
- Ciencia ambiental
Áreas temáticas:
- Miscelánea
- Física aplicada
- Métodos informáticos especiales