Comparison of early stopping criteria for neural-network-based subpixel classification
Abstract:
A neural-network-based subpixel classification is one of the most commonly used approaches to address spectral mixture problems. Neural-network subpixel-classification performance is directly related to the network-training protocols used. This letter examined early stopping criteria for network training of subpixel land-cover classification. A new stopping criterion is proposed that is based on the reduction of mean squared error (MSE) for a validation data set. We obtained excellent results by stopping the network training when the reduction of MSE between training iterations became marginal. Furthermore, the neural-network learning rate can be used as a threshold value to identify the stopping point. The approach appeared to be robust for both simulation data and actual remote-sensing data. Use of this criterion outperformed two other commonly used stopping criteria: a predefined number of training iterations and a cross-validation approach. © 2010 IEEE.
Año de publicación:
2011
Keywords:
- neural network
- Early stopping criterion
- subpixel classification
Fuente:
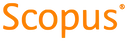
Tipo de documento:
Article
Estado:
Acceso restringido
Áreas de conocimiento:
- Aprendizaje automático
- Ciencias de la computación
Áreas temáticas:
- Métodos informáticos especiales