AI-enabled Pbkp_redictive Maintenance of Wind Generators
Abstract:
Recent policies have led to the development and deployment of renewable energy sources, introducing new operational challenges. Among those, is the problem of extended downtime of RES, such as wind turbines. In this context, it is important to minimize the downtime of renewable assets by an optimal maintenance strategy via early fault detection. As a Supervisory Control and Data Acquisition (SCADA) system is an integrated part of any production facility and collects large amounts of data, machine learning techniques can be used to detect the underlying failure patterns and notify customers of the abnormal behaviour. Thus, in this work, a novel framework based on machine learning algorithms for fault pbkp_rediction of wind farm generators is presented for an actual customer. The proposed fault prognosis methodology is tested and validated using historical data from a wind farm in Summerside, Prince Edward Island, Canada, and models are evaluated based on appropriate metrics. The results demonstrate the ability of the proposed methodology to pbkp_redict wind generator failures with a precision as high as 83%, and the viability of the proposed methodology for optimizing pbkp_redictive maintenance strategies.
Año de publicación:
2021
Keywords:
- Anomaly detection
- Machine learning
- Wind turbine
- fault prognostics
- data analytics
Fuente:
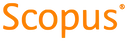
Tipo de documento:
Conference Object
Estado:
Acceso restringido
Áreas de conocimiento:
- Energía renovable
- Inteligencia artificial
Áreas temáticas:
- Física aplicada
- Ciencias de la computación
- Otras ramas de la ingeniería