Comparisons of Deep Learning Models to pbkp_redict Energy Consumption of an Educational Building
Abstract:
According to the latest United Nations Environment Programme report, in 2020, the construction and operation of buildings globally accounted for more than a third (36%) of final energy used and 37% of carbon dioxide emissions. This has given rise to research interest in energy efficiency in buildings in recent years, considering different approaches. In this work, black-box approaches based on bio-inspired techniques to pbkp_redict the energy consumption of a university building are proposed. Three artificial neural network (ANN) architectures. Feed Forward Neural Network (FFNN) such as multilayer perceptron (MLP) and recurrent neural network (RNN) such as long short-Term memory (LSTM), and gated recurrent unit (GRU). Three scenarios with different resolution timesteps and forecast horizons multi-step are tested for each architecture. The results according to the comparison of analyzed metric and computational execution times, the MLP model ranks first. These models can be very useful in pbkp_redictive control systems considered in buildings to forecast the behavior of buildings' electric load in the short term with very good precision.
Año de publicación:
2022
Keywords:
- MLP
- Artificial Neural Network
- GRU
- energy consumption forecasting
- LSTM
- deep learning
Fuente:
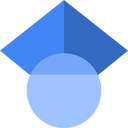
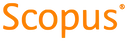
Tipo de documento:
Conference Object
Estado:
Acceso restringido
Áreas de conocimiento:
- Ingeniería energética
- Aprendizaje automático
Áreas temáticas:
- Ciencias de la computación
- Economía
- Ingeniería y operaciones afines