Compressibility of Network Opinion and Spread States in the Laplacian-Eigenvector Basis
Abstract:
Using three case studies, we examine whether snapshot data from network opinion-evolution and spread processes are compressible in the Laplacian-eigenvector basis, in the sense that each snapshot can be approximated well using a (possibly different) small set of basis vectors. The first case study is concerned with a linear consensus model that is subject to a stochastic input at an unknown location; both empirical and formal analyses are used to characterize compressibility. Second, compressibility of state snapshots for a stochastic voter model is assessed via an empirical study. Finally, compressibility is studied for state-level daily COVID-19 positivity-rate data. The three case studies indicate that state snapshots from opinion-evolution and spread processes allow terse representations, which nevertheless capture their rich propagative dynamics.
Año de publicación:
2021
Keywords:
Fuente:
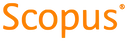
Tipo de documento:
Conference Object
Estado:
Acceso restringido
Áreas de conocimiento:
Áreas temáticas:
- Ciencias de la computación