Computational system for the detection of glioblastomas in magnetic resonance imaging using unsupervised learning
Abstract:
Conventional or advanced magnetic resonance imaging is fundamental in the evaluation of several types of tumors, including glioblastomas, given the great heterogeneity and invasiveness of these tumors, their management at present constitutes a complex challenge for clinicians and neurosur-geons. This is also true for developers of computer systems oriented to diagnostic support and monitoring. The present system is used to localize and visualize glioblastomas in 11 datasets of public use. It includes a procedure for the evaluation of certain filtering techniques in the enhancement of magnetic resonance images and, furthermore, it integrates the technique of region growth and simple link as a segmentation procedure for filtered volumes. Gaussian, median, and erosion filters are assumed as contrast enhancement techniques associated with magnetic resonance imaging. The evaluation process begins with the establishment of a set of filter configurations which depends on the values and com-binations of their input parameters. The established set of configurations is valued from the quantification of a measure of the quality of the enhancement, which is based on a scor-ing function that groups together a set of previously reported improvement metrics. From the result of the evaluation, it is concluded that the filter with the best performance corre-sponds to the median. In addition, a graphic user interface is constructed to manage the interaction between the user and the image processing algorithms.
Año de publicación:
2020
Keywords:
- Smoothing filter
- unsupervised learning
- Graphical user in-terface
- Three-dimensional visualization
- Image en-hancement
- segmentation
- Glioblastoma
- magnetic resonance
Fuente:
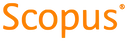
Tipo de documento:
Article
Estado:
Acceso restringido
Áreas de conocimiento:
- Aprendizaje automático
- Laboratorio médico
- Ciencias de la computación
Áreas temáticas:
- Ciencias de la computación