Considering semantics on the discovery of relations in knowledge graphs
Abstract:
Knowledge graphs encode semantic knowledge that can be exploited to enhance different data-driven tasks, e.g., query answering, data mining, ranking or recommendation. However, knowledge graphs may be incomplete, and relevant relations may be not included in the graph, affecting accuracy of these data-driven tasks. We tackle the problem of relation discovery in a knowledge graph, and devise KOI, a semantic based approach able to discover relations in portions of knowledge graphs that comprise similar entities. KOI exploits both datatype and object properties to compute the similarity among entities, i.e., two entities are similar if their datatype and object properties have similar values. KOI implements graph partitioning techniques that exploit similarity values to discover relations from knowledge graph partitions. We conduct an experimental study on a knowledge graph of TED talks with state-of-the-art similarity measures and graph partitioning techniques. Our observed results suggest that KOI is able to discover missing edges between related TED talks that cannot be discovered by state-of-the-art approaches. These results reveal that combining semantics encoded both in the similarity measures and in the knowledge graph structure, has a positive impact on the relation discovery problem.
Año de publicación:
2016
Keywords:
- Semantic similarity
- Graph partitioning
- Relation discovery
Fuente:
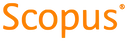
Tipo de documento:
Conference Object
Estado:
Acceso restringido
Áreas de conocimiento:
- Inteligencia artificial
- Ciencias de la computación
Áreas temáticas:
- Funcionamiento de bibliotecas y archivos