Constrained affinity matrix for spectral clustering: A basic semi-supervised extension
Abstract:
Spectral clustering has represented a good alternative in digital signal processing and pattern recognition; however a decision concerning the affinity functions among data is still an issue. In this work it is presented an extended version of a traditional multiclass spectral clustering method which employs prior information about the classified data into the affinity matrixes aiming to maintain the background relation that might be lost in the traditional manner, that is using a scaled exponential affinity matrix constrained by weighting the data according to some prior knowledge and via k-way normalized cuts clustering, results in a semi-supervised methodology of traditional spectral clustering. Test was performed over toy data classification and image segmentation and evaluated with and unsupervised performance measures (group coherence, fisher criteria and silhouette). © 2012 IEEE.
Año de publicación:
2012
Keywords:
- kernel methods
- Prior information
- Affinity matrix
- Spectral clustering
- semi-supervised analysis
Fuente:
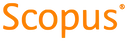
Tipo de documento:
Conference Object
Estado:
Acceso restringido
Áreas de conocimiento:
- Aprendizaje automático
- Optimización matemática
Áreas temáticas de Dewey:
- Funcionamiento de bibliotecas y archivos

Objetivos de Desarrollo Sostenible:
- ODS 9: Industria, innovación e infraestructura
- ODS 17: Alianzas para lograr los objetivos
- ODS 4: Educación de calidad
