Constructive neural networks to pbkp_redict breast cancer outcome by using gene expression profiles
Abstract:
Gene expression profiling strategies have attracted considerable interest from biologist due to the potential for high throughput analysis of hundreds of thousands of gene transcripts. Methods using artifical neural networks (ANNs) were developed to identify an optimal subset of pbkp_redictive gene transcripts from highly dimensional microarray data. The problematic of using a stepwise forward selection ANN method is that it needs many different parameters depending on the complexity of the problem and choosing the proper neural network architecture for a given classification problem is not a trivial problem. A novel constructive neural networks algorithm (CMantec) is applied in order to pbkp_redict estrogen receptor status by using data from microarrays experiments. The obtained results show that CMantec model clearly outperforms the ANN model both in process execution time as in the final prognosis accuracy. Therefore, CMantec appears as a powerful tool to identify gene signatures that pbkp_redict the ER status for a given patient. © 2010 Springer-Verlag.
Año de publicación:
2010
Keywords:
- Constructive Neural Networks
- Pbkp_redictive modelling
- Breast Cancer
- artificial neural networks
- Gene expression profiles
Fuente:
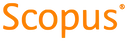
Tipo de documento:
Conference Object
Estado:
Acceso restringido
Áreas de conocimiento:
- Genética
- Aprendizaje automático
- Genética
Áreas temáticas:
- Ciencias de la computación