Contextual emotion detection on text using gaussian process and tree based classifiers
Abstract:
It is challenging for machine as well as humans to detect the presence of emotions such as sadness or disgust in a sentence without adequate knowledge about the context. Contextual emotion detection is a challenging problem in natural language processing. As the use of digital agents have increased in text messaging applications, it is essential for these agents to provide sensible responses to its users. The present work demonstrates the effectiveness of Gaussian process detecting contextual emotions present in a sentence. The results obtained are compared with Decision Tree and ensemble models such as Random Forest, AdaBoost and Gradient Boost. Out of the five models built on a small dataset with class imbalance, it has been found that Gaussian Process classifier pbkp_redicts emotions better than the other classifiers. Gaussian Process classifier performs better by taking pbkp_redictive variance into account.
Año de publicación:
2022
Keywords:
- Emotion recognition
- gradient methods
- Machine learning
- Decision Trees
- gaussian processes
Fuente:
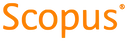
Tipo de documento:
Article
Estado:
Acceso restringido
Áreas de conocimiento:
- Aprendizaje automático
- Ciencias de la computación
Áreas temáticas:
- Ciencias de la computación