Continual Refoircement Learning Using Real-World Data for Intelligent Pbkp_rediction of SOC Consumption in Electric Vehicles
Abstract:
The accelerated migration towards electric vehicles (EV) presents several problems to solve. The main aspect is the management and pbkp_rediction of the state of charge (SOC) in real long-range routes of different variations in altitude for a more efficient energy consumption and vehicle recharge plan. This paper presents the implementation of a new algorithm for SOC estimation based on continuous learning and meta-experience replay (MER) with reservoir sample. It combines the reptile meta-learning algorithm with the experience replay technique for stabilizing the reinforcement learning. The proposed algorithm considers several important factors for the pbkp_rediction of the SOC in EV such as: speed, travel time, route altimetry, consumed battery capacity, regenerated battery capacity. A modified principal components analysis is used to reduce the dimensionality of the route altimetry data. The experimental results show an efficient estimation of the SOC values and a convergent increase in knowledge while the vehicle travels the routes.
Año de publicación:
2022
Keywords:
- Neural networks (NN)
- Electric vehicles (EV)
- Reinforcementlearning (RL)
- Meta-experience replay (MER
- principal component analysis (PCA)
- State of Charge (SOC)
Fuente:
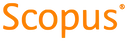
Tipo de documento:
Article
Estado:
Acceso restringido
Áreas de conocimiento:
- Aprendizaje automático
- Vehículo eléctrico
Áreas temáticas:
- Ciencias de la computación