Convolutional Neural Network for Imagine Movement Classification for Neurorehabilitation of Upper Extremities Using Low-Frequency EEG Signals for Spinal Cord Injury
Abstract:
As a result of the improvement of digital signal processing techniques and pattern recognition, it has been possible to relate brain signals with motor actions. Indeed, there are many ongoing investigations related to brain-computer interfaces that might be helpful for biomedical applications in rehabilitation procedures. This study proposes to use delta electroencephalographic signal band (0.3 Hz–3 Hz) with a classification of imagine movements using a convolutional neural network for neurorehabilitation assistant for upper limbs in patients with spinal cord injuries. This was achieved through the classification of 5 classes of movements to pbkp_redict potential imaginary movement by the training of a convolutional neural network with a specific architecture for electroencephalographic signals, EEGNet. Interpolation and independent component analysis was applied as well to optimize the training of a neural network which allowed to pbkp_redict neurophysiological motor processes with a 31% accuracy. Hence, the classification of movement-related cortical potential with convolutional neural network model opens the possibility for future Brain-Computer Interfaces applications in the biomedical field for rehabilitation processes.
Año de publicación:
2022
Keywords:
- Electroencephalogram
- Spinal cord
- Convolutional neural network
- Neurorehabilitation
Fuente:
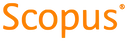
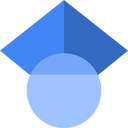
Tipo de documento:
Conference Object
Estado:
Acceso restringido
Áreas de conocimiento:
- Aprendizaje automático
- Neurología
- Ciencias de la computación
Áreas temáticas de Dewey:
- Enfermedades
- Farmacología y terapéutica
- Medicina y salud