Damage detection and diagnosis for offshore wind foundations
Abstract:
Structural health monitoring for wind turbines (WT) in remote locations, as offshore, is crucial (Presencia and Shafiee, 2018). Offshore wind farms are increasingly realized in water depths beyond 30 meters, where lattice foundations (as jacket-type) are a highly competitive substructure type (Moulas et al., 2017). In this work, a methodology for the diagnosis of structural damage in jacket-type foundations is stated by means of a small-scale structure -an experimental laboratory tower modeling an offshore-fixed jacket-type WT. In the literature, a lot of methodologies for damage detection can be found (Li et al., 2015). Among them, the vibration-based methods are one of the most prolific ones. However, they are, primarily, focused on the case of measurable input excitation and vibration response signals, with only few recent studies focused on the vibration–response–only case, the importance of which stems from the fact that in some applications the excitation cannot be imposed and often is not measurable. This work aims to contribute in this area, as the vibration excitation is given by the wind and analyzed by a convolutional neural network (CNN), with a classification accuracy result of 93 %.
Año de publicación:
2020
Keywords:
- Convolutional neural network
- Offshore wind turbine
- Data-driven
- Structural Health Monitoring
- Structural vibration
Fuente:
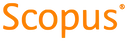
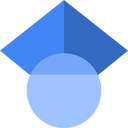
Tipo de documento:
Conference Object
Estado:
Acceso restringido
Áreas de conocimiento:
- Ingeniería estructural
- Ingeniería geotécnica
Áreas temáticas:
- Física aplicada
- Ingeniería civil
- Electricidad y electrónica