An Analysis of the Energy Consumption Forecasting Problem in Smart Buildings Using LSTM
Abstract:
This work explores the process of pbkp_redicting energy consumption in smart buildings based on the consumption of devices and appliances. Particularly, this work studies the process of data analysis and generation of pbkp_rediction models of energy consumption in Smart Buildings. Specifically, this article defines a feature engineering approach to analyze the energy consumption variables of buildings. Thus, it presents a detailed analysis of the process to build pbkp_rediction models based on time series, using real energy consumption data. According to this approach, the relationships between variables are analyzed, thanks to techniques such as Pearson and Spearman correlations and Multiple Linear Regression models. From the results obtained with these, an extraction of characteristics is carried out with the Principal Component Analysis (PCA) technique. On the other hand, the relationship of each variable with itself over time is analyzed, with techniques such as autocorrelation (simple and partial), and Autoregressive Integrated Moving Average (ARIMA) models, which help to determine the time window to generate pbkp_rediction models. Finally, pbkp_rediction models are generated using the Long Short-Term Memory (LSTM) neural network technique, taking into account that we are working with time series. This technique is useful for generating pbkp_redictive models due to its architecture and long-term memory, which allow it to handle time series very well. The generation of pbkp_rediction models is organized into three groups, differentiated by the variables that are considered as descriptors in each of them. Evaluation metrics, RMSE, MAPE, and R2 are used. Finally, the results of LSTM are compared with other techniques in different datasets.
Año de publicación:
2022
Keywords:
- Machine learning
- LSTM technique
- Energy consumption
- forecasting models
- Smart Buildings
- TIME SERIES
Fuente:
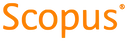
Tipo de documento:
Article
Estado:
Acceso abierto
Áreas de conocimiento:
- Energía
- Aprendizaje automático
- Energía
Áreas temáticas:
- Física aplicada
- Métodos informáticos especiales
- Ciencias de la computación