An overview of AMI data preprocessing to enhance the performance of load forecasting
Abstract:
Better understanding of actual customers' power consumption patterns is critical for improving load forecasting (LF) accuracy and efficient deployment of smart grid technologies to enhance operation, energy management, and planning of electric power systems. Though technical literature presented extensive methodologies and models to improve LF accuracy, most of them are based upon aggregated power consumption data at the system level with little or even no information regarding power consumption of different customers' classes. With the deployment of Advanced Metering Infrastructure (AMI), new energy-use information becomes available. AMI data introduces a fresh perspective to perform LF, ranging from very-short- to long- term LF at the system level, or down to the consumer level. However, one critical step to realize these benefits is to develop data management and analysis process to transform AMI data into useful information. This paper addresses the efforts involved in preparing residential customers AMI data as inputs for LF, and introduces the idea of how the preprocessed data could be further enhanced by identifying customers' consumption patterns through the application of clustering. Grouping load profiles based on consumption behavior similarities will reduce the variability of load which is going to be pbkp_redicted, and therefore, reducing the forecasting error.
Año de publicación:
2014
Keywords:
- Load Profile
- Smart Meters
- data analytics
- load forecasting
- smart Grid
- data handling
- Advanced metering infrastructure
- Clustering
- Data Mining
Fuente:
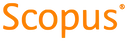
Tipo de documento:
Conference Object
Estado:
Acceso restringido
Áreas de conocimiento:
- Análisis de datos
Áreas temáticas:
- Sistemas
- El proceso político
- Física aplicada