Feature Evaluation of EMG Signals for Hand Gesture Recognition Based on Mutual Information, Fuzzy Entropy and RES Index
Abstract:
In the field of hand gesture recognition based on electromyography, the selection of features is still a matter of research because the accuracy of feature evaluation techniques depends on the distribution underling a feature space. Currently, there are a lot of kinds of features, so a selection method is required in order to find an optimal feature subset. The selection of features can be done based on either classifier methods or statistical measurement indexes. On one hand, the use of classifier methods takes considerable time, consequently, they are computationally expensive. On the other hand, the use of a statistical index allows to select features based on properties, and they are less expensive in terms of time and computational costs. In this context, this study analyzed different statistical indexes to discover optimal feature subsets and pbkp_redicts which one may have the best performance in terms of classification and recognition accuracy. For this purpose, four indexes were analyzed: the sum of RES (separation and compactness index), the sum of Fuzzy Entropies, and both of these indexes divided by the mutual information of feature subset. These index values were calculated for 10 different feature subsets obtained from nineteen time-domain features which have been investigated by many researchers for extracting useful information from an electromyography signal. The feature subsets were implemented in four classifiers (Artificial Neural Network, k-nearest Neighbors Algorithm, Support Vector Machine, and decision trees) to find the best statistical index which tries to quantify the suitableness of the feature space. Each tested index in this work was analyzed in terms of classification and recognition accuracy for each machine learning algorithm.
Año de publicación:
2021
Keywords:
- mutual information
- Feature evaluation
- Fuzzy entropy
- Hand gesture recognition
- Electromyography
- Myo armband sensor
Fuente:
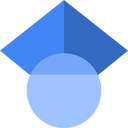
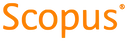
Tipo de documento:
Conference Object
Estado:
Acceso restringido
Áreas de conocimiento:
- Ciencias de la computación
- Inteligencia artificial
Áreas temáticas:
- Ciencias de la computación