Feature Extraction Analysis for Emotion Recognition from ICEEMD of Multimodal Physiological Signals
Abstract:
The emotions identification is a very complex task due to depending on multiple variables individually and as a group. They are evaluated by different criteria such as arousal, valence, and dominance mainly. Several investigations have been focused on building prediction systems. Nevertheless, this is still an open research field. The main objective of this paper is the analysis of the Improved Complementary Ensemble Empirical Mode Decomposition (ICEEMD) for feature extraction from physiological signals for emotions prediction. Physiological signals and metadata of the DEAP database were used. First, the signals were preprocessed, then three decompositions were carried out using ICEEMD, Discrete Wavelet Transform (DWT), and Maximal overlap DWT. Feature extraction was carried out using Hermite coefficients, and multiple statistic measures from IMFs, coefficients DWT, and MODWT, and signals. Then, Relief F selection algorithms were applied to reducing the dimensionality of the feature space. Finally, Linear Discriminant Classifier (LDC) and K-NN cascade, and Random Forest classifiers were tested. The different decomposition techniques were compared, and the relevant signals and measures were established. The results demonstrated the capability of ICEEMD decomposition for emotions analysis from physiological signals.
Año de publicación:
2019
Keywords:
- physiological signals
- Multimodal
- Signal processing
- Improved complementary ensemble empirical mode decomposition
- Emotion recognition
Fuente:
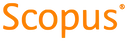
Tipo de documento:
Conference Object
Estado:
Acceso restringido
Áreas de conocimiento:
- Aprendizaje automático
- Cognición
- Ciencias de la computación
Áreas temáticas de Dewey:
- Fisiología humana
- Procesos mentales conscientes e inteligencia
- Métodos informáticos especiales

Objetivos de Desarrollo Sostenible:
- ODS 3: Salud y bienestar
- ODS 17: Alianzas para lograr los objetivos
- ODS 9: Industria, innovación e infraestructura
