Finding effective ways to (machine) learn fMRI-based classifiers from multi-site data
Abstract:
Machine learning techniques often require many training instances to find useful patterns, especially when the signal is subtle in high-dimensional data. This is especially true when seeking classifiers of psychiatric disorders, from fMRI (functional magnetic resonance imaging) data. Given the relatively small number of instances available at any single site, many projects try to use data from multiple sites. However, forming a dataset by simply concatenating the data from the various sites, often fails, due to batch effects – that is, the accuracy of a classifier learned from such a multi-site datasets, is often worse than of a classifier learned from a single site. We show why several simple, commonly used, techniques – such as including the site as a covariate, z-score normalization, or whitening – are useful only in very restrictive cases. Additionally, we propose an evaluation methodology to measure the impact of batch effects in classification studies and propose a technique for solving batch effects under the assumption that they are caused by a linear transformation. We empirically show that this approach consistently improve the performance of classifiers in multi-site scenarios, and presents more stability than the other approaches analyzed.
Año de publicación:
2018
Keywords:
- Batch effects
- Multi-site fMRI
- Machine learning
Fuente:
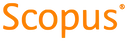
Tipo de documento:
Conference Object
Estado:
Acceso restringido
Áreas de conocimiento:
- Aprendizaje automático
- Ciencias de la computación
Áreas temáticas de Dewey:
- Ciencias de la computación