Fuzzy interval modelling based on joint supervision
Abstract:
This paper presents a new methodology for Pbkp_rediction Interval (PI) construction based on a modified Takagi-Sugeno fuzzy system trained with a joint Supervision loss function. Given a desired coverage level, this model is capable of providing pbkp_redictions of the expected value of the system along with the interval bounds. This methodology is tested by simulation experiments using a dataset containing real temperature data from a rural community in southern Chile. The proposed model was compared with a state-of-the-art Takagi-Sugeno Fuzzy Numbers model. It was shown that the Joint Supervision method manages to obtain slightly superior results to the Fuzzy Numbers approach while greatly reducing the complexity of the training loss function. Additionally, since the proposed model was trained using Particle Swarm Optimization, further performance improvements could be made by employing gradient-based optimization algorithms, since they are compatible with the Joint Supervision loss function.
Año de publicación:
2020
Keywords:
- fuzzy systems
- pbkp_rediction interval
- Joint supervision
Fuente:
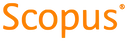
Tipo de documento:
Conference Object
Estado:
Acceso restringido
Áreas de conocimiento:
- Optimización matemática
- Lógica difusa
Áreas temáticas de Dewey:
- Ciencias de la computación