Handwritten Signature Verification System Using Convolutional Neural Network for Real-Time Applications
Abstract:
The handwritten signature is the most common method used to verify the legality of documents and plays a critical role in indicating a person’s identity. Traditionally, a person determines the validity of a signature by manually comparing it to a stored record of genuine signatures. Manual verification is time-consuming and depends on the skill of the verifier to detect forgeries. This work aims to develop a system for handwritten signature recognition using pattern recognition techniques. This work pretends to contribute to banks and companies to validate a document’s signature automatically. Handwritten signature verification systems have been approached using different methods; however, solutions with artificial intelligence stand out for their superior performance. The proposed model is based on a shallow convolutional neural network and trained with the CEDAR handwritten signature dataset. The model can recognize the handwritten signatures of 55 users, verifying their legality against forgeries with an Equal Error Rate of 1.716%, improving the performance described by other methods working on the same dataset. The developed system is lightweight and allows verification to be performed in real time. Additionally, this paper provides insightful analysis for hyperparameter optimization of the model.
Año de publicación:
2023
Keywords:
- Handwritten signature verification
- convolutional neural networks
- Writer-independent signature verification
Fuente:
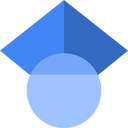
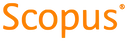
Tipo de documento:
Conference Object
Estado:
Acceso restringido
Áreas de conocimiento:
- Aprendizaje automático
- Ciencias de la computación
Áreas temáticas:
- Métodos informáticos especiales