Measuring the Impact of Data Augmentation Techniques in Lung Radiograph Classification Using a Fractional Factorial Design: A Covid-19 Case Study
Abstract:
Convolutional neural networks (CNNs) have become dominant in various computer vision tasks, obtaining state-of-the-art results in medical image analysis. Nevertheless, CNNs require large datasets to achieve high performance, which might not always be available in medical settings. Hence, different data augmentation strategies have been proposed to synthetically increase the size and diversity of a dataset. According to the state of the art, the relationship between data augmentation operations and the classification accuracy of a neural network has not been fully explored. In this work, the effect that basic augmentation techniques have in the detection of COVID-19 on chest X-Ray images is analyzed using a 2(7-1) fractional factorial experimental design. The experimental results show that zoom in and height shift operations have a significant positive effect on the accuracy, while horizontal flip operation hinders the performance. Moreover, by applying a cube plot analysis, the data augmentation operations and values that maximize the accuracy of the CNN are found. A 97% accuracy, 93% precision, and 97.7% recall scores are attained on a publicly available COVID-19 dataset using these data augmentation operations.
Año de publicación:
2022
Keywords:
- Fractional Factorial Design
- Medical Image Classification
- convolutional neural networks
- design of experiments
- Covid-19 Detection
- Image Data Augmentation
Fuente:
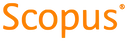
Tipo de documento:
Conference Object
Estado:
Acceso restringido
Áreas de conocimiento:
- Aprendizaje automático
- Ciencias de la computación
Áreas temáticas:
- Enfermedades
- Medicina y salud
- Ciencias de la computación