Neural Network Pbkp_rediction Interval Based on Joint Supervision
Abstract:
In this paper, a new pbkp_rediction interval model based on a joint supervision loss function for capturing the uncertainties associated with the modeled phenomenon is described. This model provides the upper and lower bounds of the pbkp_redicted values in accordance with the desired coverage probability, as well as their expected values. A benchmark problem is used to evaluate the proposed method, and a comparison with the neural network covariance method is performed. Additionally, the proposed method was applied to forecast the residential demand from a town in UK, considering the pbkp_rediction interval performance for one-day ahead. The results show that the method is able to generate an interval with narrower width than the covariance method, and maintains the coverage probability. The information provided by the pbkp_rediction interval could be used in the design of microgrid energy management systems.
Año de publicación:
2018
Keywords:
- pbkp_rediction interval
- neural network
- Joint supervision
Fuente:
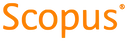
Tipo de documento:
Conference Object
Estado:
Acceso restringido
Áreas de conocimiento:
- Aprendizaje automático
- Algoritmo
Áreas temáticas:
- Programación informática, programas, datos, seguridad