Optimization of NIR calibration models for multiple processes in the sugar industry
Abstract:
The measurements of Near-Infrared (NIR) Spectroscopy, combined with data analysis techniques, are widely used for quality control in food production processes. This paper presents a methodology to optimize the calibration models of NIR spectra in four different stages in a sugar factory. The models were designed for quality monitoring, particularly °Brix and Sucrose, both common parameters in the sugar industry. A three stage optimization methodology, including pre-processing selection, feature selection and support vector machines regression metaparameters tuning, were applied to the spectral data divided by repeated cross-validation. Global models were optimized while endeavoring to ensure they are able to estimate both quality parameters with a single calibration, for the four steps of the process. The proposed models improve the pbkp_rediction for the test set (unseen data) compared to previously published models, resulting in a more accurate quality assessment of the intermediate products of the process in the sugar industry.
Año de publicación:
2016
Keywords:
- SUPPORT VECTOR MACHINES
- Agro-industry
- NIR
- Chemometrics
- Machine learning
- Calibration models
Fuente:
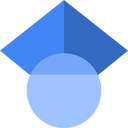
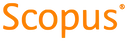
Tipo de documento:
Article
Estado:
Acceso restringido
Áreas de conocimiento:
- Optimización matemática
- Ciencia de los alimentos
- Agricultura
Áreas temáticas:
- Fabricación
- Tecnología alimentaria
- Ingeniería química