Perturbation-theory machine learning (PTML) multilabel model of the CheMBL dataset of preclinical assays for antisarcoma compounds
Abstract:
Sarcomas are a group of malignant neoplasms of connective tissue with a different etiology than carcinomas. The efforts to discover new drugs with antisarcoma activity have generated large datasets of multiple preclinical assays with different experimental conditions. For instance, the ChEMBL database contains outcomes of 37,919 different antisarcoma assays with 34,955 different chemical compounds. Furthermore, the experimental conditions reported in this dataset include 157 types of biological activity parameters, 36 drug targets, 43 cell lines, and 17 assay organisms. Considering this information, we propose combining perturbation theory (PT) principles with machine learning (ML) to develop a PTML model to pbkp_redict antisarcoma compounds. PTML models use one function of reference that measures the probability of a drug being active under certain conditions (protein, cell line, organism, etc.). In this paper, we used a linear discriminant analysis and neural network to train and compare PT and non-PT models. All the explored models have an accuracy of 89.19−95.25% for training and 89.22−95.46% in validation sets. PTML-based strategies have similar accuracy but generate simplest models. Therefore, they may become a versatile tool for pbkp_redicting antisarcoma compounds.
Año de publicación:
2020
Keywords:
Fuente:
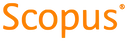
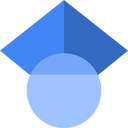
Tipo de documento:
Article
Estado:
Acceso abierto
Áreas de conocimiento:
- Aprendizaje automático
- Descubrimiento de fármacos
Áreas temáticas:
- Ciencias de la computación
- Química y ciencias afines
- Tecnología (Ciencias aplicadas)