Pbkp_rediction interval modeling tuned by an improved teaching learning algorithm applied to load forecasting in microgrids
Abstract:
In recent years there has been growing interest in pbkp_rediction models for non-conventional energy sources and demand in electrical systems because of the increasing use of renewable energy sources. The pbkp_rediction interval models proposed in this paper are validated using local load data from a real-life micro grid in Huatacondo, Chile. The micro grid operates with an energy management system (EMS), which dispatches distributed generators based on unit commitment, minimizing generation costs. The relevant inputs for the EMS are pbkp_redictions of the consumption and the available amount of renewable resources. In this paper a linear and a Takagi-Sugeno fuzzy model are proposed and they are used to construct a pbkp_rediction interval that includes a representation of the uncertainties. The model parameters are identified such that they minimize a multi-objective cost function that not only includes the error but also the width of the pbkp_rediction interval and its coverage probability. The resulting parameter identification is a complex non-convex problem. An Improved Teaching Learning Based Optimization (ITLBO) algorithm is proposed in order to solve the problem. This method is compared with a Particle Swarm Optimization procedure for a benchmark problem, showing that both algorithms find similar results. ITLBO is used to identify the load pbkp_rediction models. These models are used to pbkp_redict load up to two days ahead. Both models succeed in accomplish the design objectives.
Año de publicación:
2015
Keywords:
Fuente:
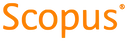
Tipo de documento:
Conference Object
Estado:
Acceso restringido
Áreas de conocimiento:
- Optimización matemática
- Aprendizaje automático
Áreas temáticas:
- Métodos informáticos especiales
- Ciencias de la computación
- Economía de la tierra y la energía