Predictive modeling of groundwater nitrate pollution using Random Forest and multisource variables related to intrinsic and specific vulnerability: A case study in an agricultural setting (Southern Spain)
Abstract:
Watershed management decisions need robust methods, which allow an accurate predictive modeling of pollutant occurrences. Random Forest (RF) is a powerful machine learning data driven method that is rarely used in water resources studies, and thus has not been evaluated thoroughly in this field, when compared to more conventional pattern recognition techniques key advantages of RF include: its non-parametric nature; high predictive accuracy; and capability to determine variable importance. This last characteristic can be used to better understand the individual role and the combined effect of explanatory variables in both protecting and exposing groundwater from and to a pollutant.In this paper, the performance of the RF regression for predictive modeling of nitrate pollution is explored, based on intrinsic and specific vulnerability assessment of the Vega de Granada aquifer. The applicability of this new machine learning technique is demonstrated in an agriculture-dominated area where nitrate concentrations in groundwater can exceed the trigger value of 50. mg/L, at many locations. A comprehensive GIS database of twenty-four parameters related to intrinsic hydrogeologic proprieties, driving forces, remotely sensed variables and physical-chemical variables measured in "situ", were used as inputs to build different predictive models of nitrate pollution. RF measures of importance were also used to define the most significant predictors of nitrate pollution in groundwater, allowing the establishment of the pollution sources (pressures).The potential of RF for generating a vulnerability map to nitrate pollution is assessed considering multiple criteria related to variations in the algorithm parameters and the accuracy of the maps. The performance of the RF is also evaluated in comparison to the logistic regression (LR) method using different efficiency measures to ensure their generalization ability. Prediction results show the ability of RF to build accurate models with strong predictive capabilities. © 2014 Elsevier B.V.
Año de publicación:
2014
Keywords:
- random forest
- Nitrates
- vulnerability assessment
- Machine learning techniques
- Groundwater
Fuente:
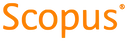
Tipo de documento:
Article
Estado:
Acceso restringido
Áreas de conocimiento:
- Recursos hídricos
- Aprendizaje automático
- Hidrología
Áreas temáticas de Dewey:
- Geología, hidrología, meteorología
- Técnicas, equipos y materiales
- Ingeniería sanitaria

Objetivos de Desarrollo Sostenible:
- ODS 6: Agua limpia y saneamiento
- ODS 15: Vida de ecosistemas terrestres
- ODS 2: Hambre cero
