A Stochastic Dual Dynamic Programming Framework for Multiscale MPC<sup>⁎</sup>
Abstract:
We derive and interpret stochastic dual dynamic programming (SDDP) from the perspective of MPC to argue that SDDP provides a scalable approach to handle complex MPC applications with uncertainties evolving over long time horizons and with fine time resolutions. Scalability is enabled by the use of a nested cutting-plane scheme, which uses forward and backward sweeps along the time horizon to adaptively construct and refine cost-to-go functions. SDDP can also select random paths on-the-fly, thus avoiding the exponential complexity associated with the construction of scenario trees and enabling the construction of statistical performance bounds. Our work seeks to establish connections between SDDP and MPC that allow us to obtain performance bounds for deterministic and stochastic MPC and to propose new MPC schemes that can deal with multiple timescales. We demonstrate the developments using a stationary battery system that participates in multiscale energy markets.
Año de publicación:
2018
Keywords:
- stochastic dynamic programming
- multiscale
- duality
- MPC
- energy systems
Fuente:
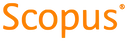
Tipo de documento:
Conference Object
Estado:
Acceso abierto
Áreas de conocimiento:
- Optimización matemática
- Optimización matemática
Áreas temáticas:
- Programación informática, programas, datos, seguridad
- Ingeniería y operaciones afines
- Métodos informáticos especiales