Prototype reduction algorithms comparison in nearest neighbor classification for sensor data: Empirical study
Abstract:
This work presents a comparative study of prototype selection (PS) algorithms. Such a study is done over data-from-sensor acquired by an embedded system. Particularly, five flexometers are used as sensors, which are located inside a glove aimed to read sign language. Measures were taken to quantify the balance between classification performance and reduction training set data (QCR) with k neighbors equal to 3 and 1 to force the classifier (kNN) to the maximum. Two tests were used: (a)the QCR performance and (b) the embedded system decision in real proves. As result the Random Mutation Hill Climbing (RMHC) algorithm is considered the best option to choose in this data type with removed instances at 87% and classification performance at 82% in software tests, also the classifier kNN must be with k=3 to improve the classification performance. In a real situation, with the algorithm implemented. The system makes correct decisions at 81% with 5 persons doing sign language in real time.
Año de publicación:
2017
Keywords:
- prototype selection
- sensor data
- Knn
Fuente:
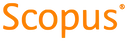
Tipo de documento:
Conference Object
Estado:
Acceso restringido
Áreas de conocimiento:
- Aprendizaje automático
- Algoritmo
Áreas temáticas:
- Ciencias de la computación