Studying MOEAs dynamics and their performance using a three compartmental model
Abstract:
The road to a better design of multi- and many-objective evolutionary algorithms requires a deeper understanding of their behavior. A step on this road has recently been taken with the proposal of compartmental models to study population dynamics. In this work, we push this step further by introducing a new set of features that we link with algorithm performance. By tracking the number of newly discovered Pareto Optimal (PO) solutions, the previously-found PO solutions and the remaining non-PO solutions, we can track the algorithm progression. By relating these features with a performance measure, such as the hypervolume, we can analyze their relevance for algorithm comparison. This study considers out-of-the-box implementations of recognized multi- and many-objective optimizers belonging to popular classes such as conventional Pareto dominance, extensions of dominance, indicator, and decomposition based approaches. In order to generate training data for the compartmental models, we consider multiple instances of MNK-landscapes with different numbers of objectives.
Año de publicación:
2018
Keywords:
- Working principles of evolutionary computing
- Genetic Algorithms
- multi-objective optimization
- empirical study
Fuente:
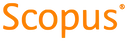
Tipo de documento:
Conference Object
Estado:
Acceso restringido
Áreas de conocimiento:
- Optimización matemática
- Optimización matemática
- Modelo matemático
Áreas temáticas de Dewey:
- Ciencias de la computación

Objetivos de Desarrollo Sostenible:
- ODS 9: Industria, innovación e infraestructura
- ODS 17: Alianzas para lograr los objetivos
- ODS 4: Educación de calidad
