Studying compartmental models interpolation to estimate MOEAS population size
Abstract:
Dynamical compartmental models capture the population dynamics of Multi-objective Optimization Evolutionary Algorithms. In these models, solutions at each generation are classified in compartments according to Pareto dominance. The size of each model compartment is affected by the other components and changes throughout the generations. Once the dynamics are learned, given the composition of each compartment for the initial population, the model can predict their changes in future generations. In this work, we use these models to select an appropriate population size for a given budget of fitness evaluations. We first learn models parameters for a subset of population sizes and then extract new models from existing ones by spline interpolation. The proposed method allows the investigation of population sizes in-between the ones for which data is available. We verify the proposed method using MNK-Landscapes with twenty bits and one epistatic interaction, running the Adaptive ϵ-Sampling ϵ-Hood MOEA on several population configurations.
Año de publicación:
2019
Keywords:
- multi-objective optimization
- empirical study
- Working principles of evolutionary computing
- Genetic Algorithms
Fuente:
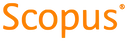
Tipo de documento:
Conference Object
Estado:
Acceso restringido
Áreas de conocimiento:
- Modelo matemático
- Optimización matemática
Áreas temáticas de Dewey:
- Ciencias de la computación
- Ciencias Naturales y Matemáticas
- Probabilidades y matemática aplicada

Objetivos de Desarrollo Sostenible:
- ODS 9: Industria, innovación e infraestructura
- ODS 17: Alianzas para lograr los objetivos
- ODS 4: Educación de calidad
