Visualization as Intermediate Representations (VLAIR) for Human Activity Recognition
Abstract:
Ambient, binary, event-driven sensor data is useful for many human activity recognition applications such as smart homes and ambient-assisted living. These sensors are privacy-preserving, unobtrusive, inexpensive and easy to deploy in scenarios that require detection of simple activities such as going to sleep, and leaving the house. However, classification performance is still a challenge, especially when multiple people share the same space or when different activities take place in the same areas. To improve classification performance we develop what we call a Visualization as Intermediate Representations (VLAIR) approach. The main idea is to re-represent the data as visualizations (generated pixel images) in a similar way as how visualizations are created for humans to analyze and communicate data. Then we can feed these images to a convolutional neural network whose strength resides in extracting effective visual features. We have tested five variants (mappings) of the VLAIR approach and compared them to a collection of classifiers commonly used in classic human activity recognition. The best of the VLAIR approaches outperforms the best baseline, with strong advantage in recognising less frequent activities and distinguishing users and activities in common areas. We conclude the paper with a discussion on why and how VLAIR can be useful in human activity recognition scenarios and beyond.
Año de publicación:
2020
Keywords:
- convolutional neural networks
- Smart homes
- Intermediate representations
- Information Visualization
- Human activity recognition
Fuente:
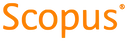
Tipo de documento:
Conference Object
Estado:
Acceso restringido
Áreas de conocimiento:
- Aprendizaje automático
- Ciencias de la computación
Áreas temáticas de Dewey:
- Métodos informáticos especiales
- Procesos sociales
- Fisiología humana