Weighted Hausdorff Distance Loss as a Function of Different Metrics in Convolutional Neural Networks for Ladybird Beetle Detection
Abstract:
This work compares five different distance metrics (i.e., Euclidean, Chebyshev, Manhattan, Mahalanobis, and Canberra) implemented in the weighted Hausdorff distance (WHD) as part of the loss function during the training and validation of a fully convolutional neural network (FCNN) model for detecting ladybird beetle specimens. The FCNN-based detector was trained and validated using a ten-fold cross-validation method on a database composed of 2,633 wildlife images with ladybird beetles. The obtained results highlighted the Chebyshev metric as the top performer given a diverse dataset as ours. This metric scored the highest values in three out of four validation metrics (i.e., precision, recall, and F1-score). The nature of this metric allows substantial space for minimizing the cost function along the FCNN training step. Euclidean and Manhattan distances also provide good performance based on our validation metrics, while Mahalanobis and Canberra distances are not suitable for detecting of ladybird beetles.
Año de publicación:
2022
Keywords:
- heat map
- Ladybird beetle detection
- weighted Hausdorff distance
- deep learning
- fully convolutional neural network
Fuente:
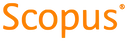
Tipo de documento:
Conference Object
Estado:
Acceso restringido
Áreas de conocimiento:
- Aprendizaje automático
- Ciencias de la computación
Áreas temáticas de Dewey:
- Métodos informáticos especiales
- Programación informática, programas, datos, seguridad
- Ciencias de la computación

Objetivos de Desarrollo Sostenible:
- ODS 15: Vida de ecosistemas terrestres
- ODS 2: Hambre cero
- ODS 9: Industria, innovación e infraestructura
