Assessment of Multispectral Vegetation Features for Digital Terrain Modeling in Forested Regions
Abstract:
Bare-earth extraction in forested regions has been considered challenging because of the lack of ground point information. In these regions, vision systems cannot capture any information about ground points under the canopy. Thus, the challenge of generating a digital terrain model by cameras increases. Nevertheless, one might alleviate ground filtering using vegetation's features (e.g., chlorophyll). In this regard, this article evaluated two machine-learning approaches [i.e., conditional random field (CRF), artificial neural network (ANN)] for generating digital terrain models when biophysical or biochemical features of vegetation are given. Terrain models were generated from multispectral image-based point clouds. A fivefold cross-validation methodology evaluated the CRF and ANN. The point clouds were retrieved from two study areas at different illumination and flight altitudes. Vegetation features were computed as vegetation indices from the multispectral point clouds. Results suggested that by using these indices, the classification of ground points could be enhanced. In particular, the vegetation indices that yielded the best outcomes were normalized difference vegetation index, green NDVI, and modified chlorophyll absorption reflectance index. Moreover, it was shown that CRF generates elevation models more smoothly than a triangular irregular network method. Thus, a CRF could be promising for classifying ground points in forested regions using geometric and vegetation features from a photogrammetric point cloud.
Año de publicación:
2022
Keywords:
- Conditional random field (CRF)
- Digital terrain model
- vegetation indices
- multispectral
Fuente:
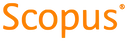
Tipo de documento:
Article
Estado:
Acceso restringido
Áreas de conocimiento:
- Sensores remotos
- Sensores remotos
- Visión por computadora
Áreas temáticas:
- Agricultura y tecnologías afines
- Técnicas, equipos y materiales
- Huertos, frutas, silvicultura