A semi-supervised learning approach to study the energy consumption in smart buildings
Abstract:
In this work, we use the semi-supervised LAMDA-HSCC algorithm for characterizing the energy consumption in smart buildings, which can work with labeled and unlabeled data. Particularly, it uses the LAMDA-RD approach for the clustering problem and the LAMDA-HAD approach for the classification problem. Additionally, this algorithm uses three submodels for merging, partition groups (classes/cluster) and migrating individuals from a group to another. For the performance evaluation, several datasets of energetic consumption are used, with different percent of labeled data, showing very encouraging results according to two metrics in the semi-supervised context.
Año de publicación:
2021
Keywords:
- semi-supervised learning
- LAMDA
- Multivariate Data Analysis
- Energetic Consumption
Fuente:
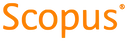
Tipo de documento:
Conference Object
Estado:
Acceso restringido
Áreas de conocimiento:
- Aprendizaje automático
- Energía
- Simulación por computadora
Áreas temáticas de Dewey:
- Métodos informáticos especiales
- Física aplicada
- Ciencias de la computación

Objetivos de Desarrollo Sostenible:
- ODS 7: Energía asequible y no contaminante
- ODS 11: Ciudades y comunidades sostenibles
- ODS 9: Industria, innovación e infraestructura
