A synthetic Data Generator for Smart Grids based on the Variational-Autoencoder Technique and Linked Data Paradigm
Abstract:
In a smart environment like the smart grids, it is necessary to have knowledge models that allow solving emerging problems. However, large datasets are required to automatically create these models, which in the vast majority of cases are not available. Therefore, in these environments is essential to have a data generator for each context. In this paper, we propose a synthetic data generation system based on the variational autoencoder (VAE) technique and linked data paradigm, to create larger datasets from small datasets acting as samples. Specifically, a Linked Data-based dataset extractor is proposed, which allows obtaining samples of data in a particular context. Then, a VAE is trained with these samples of data available in a specific context, to learn the latent distribution that characterizes the dataset, allowing the production of new records that are similar to those of the original dataset. Finally, several case studies in the field of energy management are carried out. In one of them, the process that follows our approach is described in detail; and then, another 3 more are considered to evaluate its ability to automate the data generation process for smart energy management. The results show how our synthetic data generation system can be used to obtain synthetic datasets in different energy contexts.
Año de publicación:
2022
Keywords:
- Variational autoencoders
- Synthetic Data Generator
- Linked data
- deep learning
- smart Grid
Fuente:
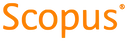
Tipo de documento:
Conference Object
Estado:
Acceso restringido
Áreas de conocimiento:
- Ciencias de la computación
- Aprendizaje automático
- Simulación por computadora
Áreas temáticas de Dewey:
- Ciencias de la computación

Objetivos de Desarrollo Sostenible:
- ODS 7: Energía asequible y no contaminante
- ODS 13: Acción por el clima
- ODS 9: Industria, innovación e infraestructura
