Closed state model for understanding the dynamics of MOEAs
Abstract:
This work proposes the use of simple closed state models to capture, analyze and compare the dynamics of multi- and many-objective evolutionary algorithms. Two- and three-state models representing the composition of the instantaneous population are described and learned for representatives of the major approaches to multi-objective optimization, i.e. dominance, extensions of dominance, decomposition, and indicator algorithms. The model parameters are trained from data obtained running the algorithms with various population sizes on enumerable MNK-landscapes with 3, 4, 5 and 6 objectives. We show ways to interpret and use the model parameter values in order to analyze the population dynamics according to selected features. For example, we are interested in knowing how parameter values change for a given population size with the increase of the number of objectives. We also show a graphical representation capturing in one graph how the parameters magnitude and sign relate to the connections between states.
Año de publicación:
2017
Keywords:
- Genetic Algorithms
- multi-objective optimization
- Working principles of evolutionary computing
- empirical study
Fuente:
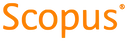
Tipo de documento:
Conference Object
Estado:
Acceso restringido
Áreas de conocimiento:
- Algoritmo
- Algoritmo
- Evolución
Áreas temáticas:
- Métodos informáticos especiales