Combining Transformer Embeddings with Linguistic Features for Complex Word Identification
Abstract:
Identifying which words present in a text may be difficult to understand by common readers is a well-known subtask in text complexity analysis. The advent of deep language models has also established the new state-of-the-art in this task by means of end-to-end semi-supervised (pre-trained) and downstream training of, mainly, transformer-based neural networks. Nevertheless, the usefulness of traditional linguistic features in combination with neural encodings is worth exploring, as the computational cost needed for training and running such networks is becoming more and more relevant with energy-saving constraints. This study explores lexical complexity pbkp_rediction (LCP) by combining pre-trained and adjusted transformer networks with different types of traditional linguistic features. We apply these features over classical machine learning classifiers. Our best results are obtained by applying Support Vector Machines on an English corpus in an LCP task solved as a regression problem. The results show that linguistic features can be useful in LCP tasks and may improve the performance of deep learning systems.
Año de publicación:
2023
Keywords:
- Lexical complexity pbkp_rediction
- linguistic features
- pre-trained large language models
- features fusion
Fuente:
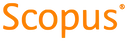
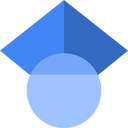
Tipo de documento:
Article
Estado:
Acceso abierto
Áreas de conocimiento:
- Ciencias de la computación
Áreas temáticas:
- Lengua