Comparative Study of Artificial Neural Network Based Channel Equalization Methods for mmWave Communications
Abstract:
In this paper, we compare two artificial neural networks (ANNs) approaches designed to perform channel equalization for millimeter-wave (mmWave) signals operating in the 28 GHz frequency band. We used an in-house deterministic Three-Dimensional Ray-Launching (3D-RL) code to simulate the spatial structure of mmWave channels considering the material properties of the obstacles within the scenario at the frequency under analysis. We performed offline training of a multilayer perceptron (MLP) neural network with the simulated mmWave channels to equalize the received signal. We also performed online training of an extreme learning machine (ELM) neural network to directly get the equalized symbols at the receiver, given as input the received mmWave signal. The ANN solutions were tested in terms of the achievable spectral efficiency, bit error rate, and time to process. We compared the ANN techniques to the minimum mean square error and the zero-forcing equalizers, considering an orthogonal frequency-division multiplexing communication based on the 5G New Radio standard. We present numerical results on the performance of the proposed ANNs and show that the ELM strategy outperforms the MLP method, requiring significantly less processing time than the reviewed equalization methods.
Año de publicación:
2021
Keywords:
- Multilayer Perceptron
- mmWave communications
- elm
- OFDM
- 5G and beyond
- channel equalization
Fuente:
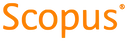
Tipo de documento:
Article
Estado:
Acceso abierto
Áreas de conocimiento:
- Red neuronal artificial
- Ciencias de la computación
Áreas temáticas:
- Ciencias de la computación