Analyzing Feature Importance for a Pbkp_redictive Undergraduate Student Dropout Model
Abstract:
Worldwide, one of the main concerns of universities is to reduce the dropout rate. Several initiatives have been taken to avoid this problem; however, it is essential to recognize at-risk students as early as possible. This article is an extension of a previous study that proposed a pbkp_redictive model to identify students at risk of dropout from the beginning of their university degree. The new contribution is the analysis of the feature importance for dropout segmented by faculty, degree program, and semester in the different pbkp_redictive models. In addition, we propose a dropout model based on faculty characteristics to try to infer the dropout based on faculty features. We used data of 30,576 students enrolled in a Higher Education Institution ranging from years 2000 to 2020. The findings indicate that the variables related to Grade Point Average(GPA), socioeconomic factor, and a pass rate of courses taken have a more significant impact on the model, regardless of the semester, faculty, or program. Additionally, we found a significant difference in the pbkp_redictive power between Science, Technology, Engineering, and Mathematics (STEM) and humanistic programs.
Año de publicación:
2023
Keywords:
- features importance
- dropout model
- Data Mining
- learning analytics
Fuente:
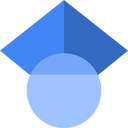
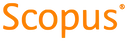
Tipo de documento:
Article
Estado:
Acceso abierto
Áreas de conocimiento:
- Aprendizaje automático
- Educación superior
Áreas temáticas:
- Escuelas y sus actividades; educación especial
- Programación informática, programas, datos, seguridad
- Funcionamiento de bibliotecas y archivos