Insights in Learners' Behaviour and Early Dropout Detection based on Coursera MOOCs
Abstract:
Increased utilization of distance learning makes the creation of effective learning tools ever more important. However, while Massive Open Online Courses (MOOCs) are a popular online learning tool, they often suffer from high user attrition. This paper investigated this effect for 6 different MOOCs with more than 35 thousand users, using AdaBoosted decision trees to create a model which was then used for the pbkp_rediction of dropouts, as well as the calculation of feature importance scores. The resulting model generally scored high accuracy scores which were plotted for each course, and feature importance scores were especially high for the features encompassing the number of user requests, the user's total active time and the average time between clicks. Furthermore, the paper explored the results of the inclusion of forum data in this setting. While the forum features did not lead to a major increase in model accuracy, there was a statistical difference between the number of forum interactions for those who completed the MOOCs and those who did not, which opens up possibilities for future research into utilization of forum interaction data, and forums in MOOCs in general.
Año de publicación:
2021
Keywords:
- Mooc
- Dropout Detection
- Coursera
Fuente:
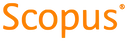
Tipo de documento:
Conference Object
Estado:
Acceso restringido
Áreas de conocimiento:
- Tecnología educativa
Áreas temáticas:
- Educación
- Escuelas y sus actividades; educación especial
- Educación de adultos