Low Resolution Electroencephalographic-Signals-Driven Semantic Retrieval: Preliminary Results
Abstract:
Nowadays, there exist high interest in the brain-computer interface (BCI) systems, and there are multiple approaches to developing them. Lexico-semantic (LS) classification from Electroencephalographic (EEG) signals is one of them, which is an open and few explored research field. The LS depends on the creation of the concepts of each person and its context. Therefore, it has not been demonstrated a universal fingerprint of the LS either the spatial location in the brain, which depends on the variability the brain plasticity and other changes throughout the time. In this study, an analysis of LS from EEG signals was carried out. The Emotiv Epoc+ was used for the EEG acquisition from three participants reading 36 different words. The subjects were characterized throughout two surveys (Becks depression, and emotion test) for establishing their emotional state, depression, and anxiety levels. The signals were processed to demonstrate semantic category and for decoding individual words (4 pairs of words were selected for this study). The methodology was executed as follows: first, the signals were pre-processed, decomposed by sub-bands (δ, θ, α, β, and γ ) and standardized. Then, feature extraction was applied using linear and non-linear statistical measures, and the Discrete Wavelet Transform calculated from EEG signals, generating the feature space termed set-1. Also, the principal component analysis was applied to reduce the dimensionality, generating the feature space termed set-2. Finally, both sets were tested independently by multiple classifiers based on the support vector machine and k- nearest neighbor. These were validated using 10-fold cross-validation achieving results upper to 95% of accuracy which demonstrated the capability of the proposed mechanism for decoding LS from a reduced number of EEG signals acquired using a portable system of acquisition.
Año de publicación:
2019
Keywords:
- Electroencephalographic signal
- Machine learning
- Signal processing
- Semantic retrieval
- Semantic category
Fuente:
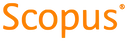
Tipo de documento:
Conference Object
Estado:
Acceso restringido
Áreas de conocimiento:
- Inteligencia artificial
- Ciencias de la computación
- Neuropsicología
Áreas temáticas de Dewey:
- Funcionamiento de bibliotecas y archivos

Objetivos de Desarrollo Sostenible:
- ODS 3: Salud y bienestar
- ODS 4: Educación de calidad
- ODS 9: Industria, innovación e infraestructura
